- I data lake sono vasti depositi di dati grezzi e non strutturati, che offrono flessibilitร e scalabilitร per l'archiviazione di grandi volumi di informazioni. Sono ideali per l'esplorazione e per potenziali casi d'uso futuri.ย ย ย ย
- I data warehouse sono archivi strutturati di dati elaborati, ottimizzati per l'interrogazione e l'analisi. Sono progettati per la business intelligence e il reporting, fornendo un'unica fonte di veritร per il processo decisionale.ย ย ย ย
- Sia i data lake che i data warehouse hanno i loro punti di forza e di debolezza. Spesso รจ vantaggioso un approccio ibrido, in cui i dati grezzi vengono inizialmente archiviati in un data lake per l'esplorazione, e poi i dati accuratamente selezionati vengono spostati in un data warehouse per analisi e reportistica avanzate.ย
Laghi di dati e magazzini di dati: Le pietre miliari della produzione modernaย
L'industria manifatturiera sta vivendo una rivoluzione dei dati. Grazie ai progressi della tecnologia, le fabbriche stanno generando volumi di dati senza precedenti provenienti da macchine, sensori e operazioni. Per sfruttare questi dati e promuovere l'efficienza operativa, l'innovazione e il processo decisionale, i produttori si rivolgono sempre piรน spesso a data lake e data warehouse.ย
ย
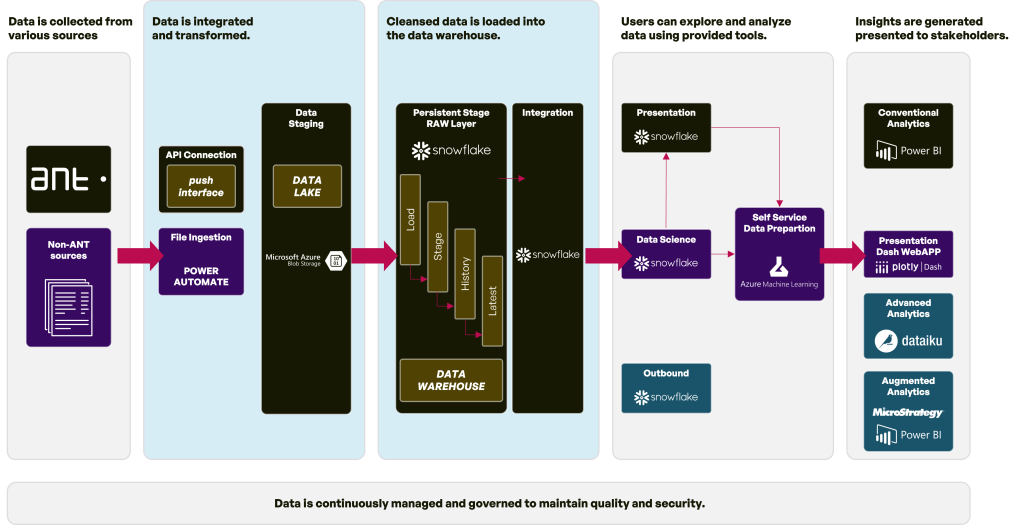
ย
Lago di dati: Un serbatoio di dati grezziย
Un data lake รจ un repository centralizzato che archivia grandi quantitร di dati grezzi nel loro formato nativo. A differenza di un data warehouse, che si concentra sui dati strutturati e sulla business intelligence, un data lake รจ progettato per contenere una varietร di tipi di dati, compresi quelli strutturati, semi-strutturati e non strutturati.ย ย ย ย
Caratteristiche principali di un Data Lakeย
Archiviazione di dati grezzi: I dati vengono memorizzati nel loro formato originale senza alcuna elaborazione o trasformazione iniziale.ย ย ย ย
- Scalabilitร : ร in grado di gestire enormi volumi di dati, che crescono in base alle esigenze.ย ย ย ย
- Varietร : Accoglie diversi tipi di dati, dal testo alle immagini, dai video ai dati dei sensori.ย ย ย ย
- Velocitร : Consente di ingerire rapidamente i dati da varie fonti.ย ย ย ย
- Flessibilitร : Supporta diversi strumenti di analisi e casi d'uso.ย
Data Warehouse, cos'รจ?ย
D'altra parte, un magazzino dati รจ un archivio centralizzato che memorizza dati integrati provenienti da piรน fonti per l'analisi e il reporting. In condizioni di produzione, l'implementazione di un data warehouse offre diversi vantaggi:ย
-
- Miglioramento del processo decisionale: Consente un migliore processo decisionale fornendo l'accesso a dati storici e in tempo reale per l'analisi.ย
-
- Efficienza migliorata: Semplifica i processi di gestione dei dati, riducendo il tempo dedicato alla loro raccolta e preparazione.ย
-
- Maggiore visibilitร : Offre una visione completa delle operazioni, facilitando il monitoraggio e il controllo.ย
-
- Qualitร dei dati: Migliora la qualitร dei dati attraverso processi di pulizia e integrazione dei dati.ย
-
- Riduzione dei costi: Aiuta a identificare le opportunitร di risparmio e a ottimizzare l'allocazione delle risorse.ย
-
- Analisi predittiva: Supporta l'analisi predittiva e le previsioni per anticipare le tendenze e prendere decisioni proattive.ย
Data Lake vs. Data Warehouseย
Data Lake:ย
-
- Definizione: Un data lake รจ un vasto bacino di dati grezzi, spesso non strutturati, che consente un'esplorazione e un'analisi flessibile.ย
-
- Caratteristiche:ย
-
- Tipo di dati: Fonti di dati grezzi, non strutturati e diversi.ย
-
- Utilizzo: Ideale per archiviare grandi volumi di dati nel loro formato nativo per una futura elaborazione.ย
-
- Flessibilitร : Supporta vari tipi e formati di dati senza schemi predefiniti.ย
-
- Pro:ย
-
- Scalabilitร : Puรฒ gestire enormi quantitร di dati.ย
-
- Flessibilitร : Accoglie diversi tipi e formati di dati.ย
-
- Contro:ย
-
- Complessitร : Richiede un'attenta governance e gestione dei dati.ย
Magazzino dati:ย
-
- Definizione: Un data warehouse รจ un archivio strutturato di dati elaborati e organizzati, utilizzati per la reportistica e l'analisi.ย
-
- Caratteristiche:ย
-
- Tipo di dati: Dati strutturati ed elaborati ottimizzati per l'interrogazione e l'analisi.ย
-
- Utilizzo: Progettato per la business intelligence e i processi decisionali.ย
-
- Schema: I dati sono organizzati in schemi predefiniti per un accesso rapido.ย
-
- Pro:ย
-
- Prestazioni: Ottimizzato per l'elaborazione rapida delle query.ย
-
- Coerenza: Fornisce un'unica fonte di veritร per i rapporti.ย
-
- Contro:ย
-
- Scalabilitร : Puรฒ trovarsi di fronte a problemi di gestione di volumi di dati non strutturati o di grandi dimensioni.ย
Confronto con il Data Warehouseย
Sebbene sia i data lake che i data warehouse immagazzinino dati, i loro scopi e approcci differiscono:ย
Caratteristicaย | Lago di datiย | Magazzino datiย |
Dataย | Grezzo, non strutturato, semistrutturatoย | Strutturato, elaboratoย |
Focusย | Varietร e volumeย | Analisi e reportisticaย |
Accessoย | Accesso diretto per l'esplorazioneย | Ottimizzato per le queryย |
Costoย | Costi iniziali piรน bassi, costi di lavorazione piรน elevatiย | Costi iniziali piรน elevati, costi di lavorazione inferioriย |
Come lavorano insieme data lake e data warehouse?ย
Sebbene i data lake e i data warehouse abbiano scopi diversi, spesso sono complementari. Molte aziende adottano un approccio ibrido, utilizzando un data lake per l'ingestione e l'esplorazione iniziale dei dati e spostando poi i dati accuratamente curati in un data warehouse per analisi e reportistica avanzate. Combinando efficacemente questi due approcci, le aziende produttrici possono sbloccare il pieno potenziale dei loro dati, promuovendo l'eccellenza operativa e ottenendo un vantaggio competitivo.ย
Quando considerare data lake e data warehouse?ย
La scelta tra un data lake e un data warehouse dipende spesso dalle esigenze specifiche di un'azienda manifatturiera. Se avete bisogno di una soluzione flessibile ed economica per archiviare grandi quantitร di dati grezzi e non strutturati per analisi esplorative e potenziali casi d'uso futuri, un data lake รจ la scelta ideale. Tuttavia, se l'obiettivo principale รจ fornire un accesso rapido, coerente e affidabile ai dati strutturati per la business intelligence e il reporting, รจ piรน adatto un data warehouse. In molti casi, un approccio ibrido che combina entrambe le soluzioni offre il meglio dei due mondi, consentendo ai produttori di archiviare ed elaborare i dati in modo efficiente, supportando al contempo varie esigenze analitiche.ย
Cosa c'รจ dopo?ย
I data lake e i data warehouse sono componenti essenziali di una Enterprise Data Platform (EDP). Tuttavia, rappresentano solo una parte di questa architettura completa. Una EDP integra diverse fonti di dati, processi e tecnologie per creare una piattaforma unificata per il processo decisionale basato sui dati. Per comprendere appieno la potenza di una EDP, esplorate i capitoli seguenti per approfondire le sue caratteristiche. analisi dei dati.ย
Per saperne di piรน
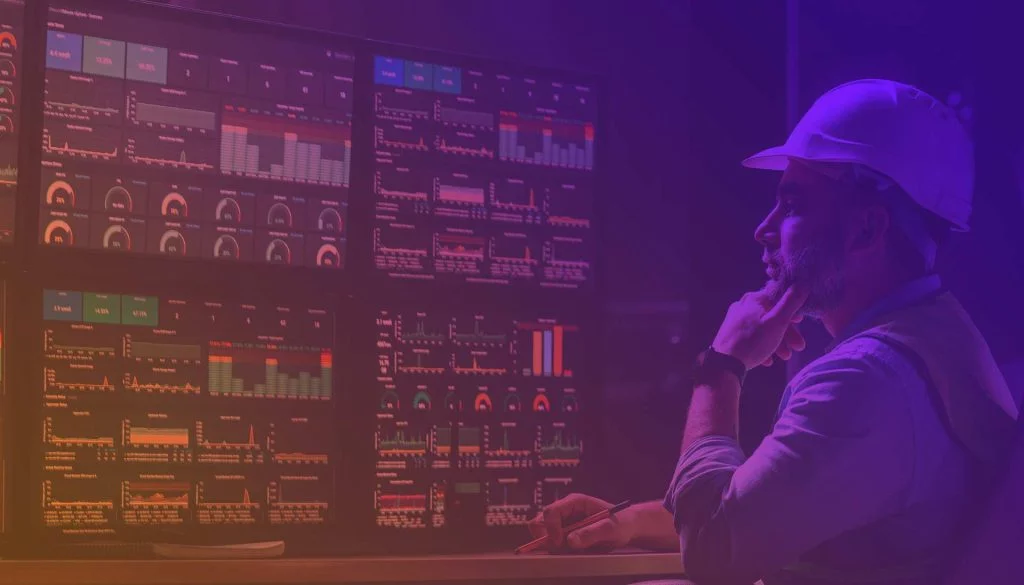
Data warehouse vs data lake, quali sono le differenze?ย
Data Lake e Data Warehouse: Le pietre miliari della produzione moderna L'industria manifatturiera sta vivendo una rivoluzione dei dati. Grazie ai progressi della tecnologia, le fabbriche stanno generando dati senza precedenti.
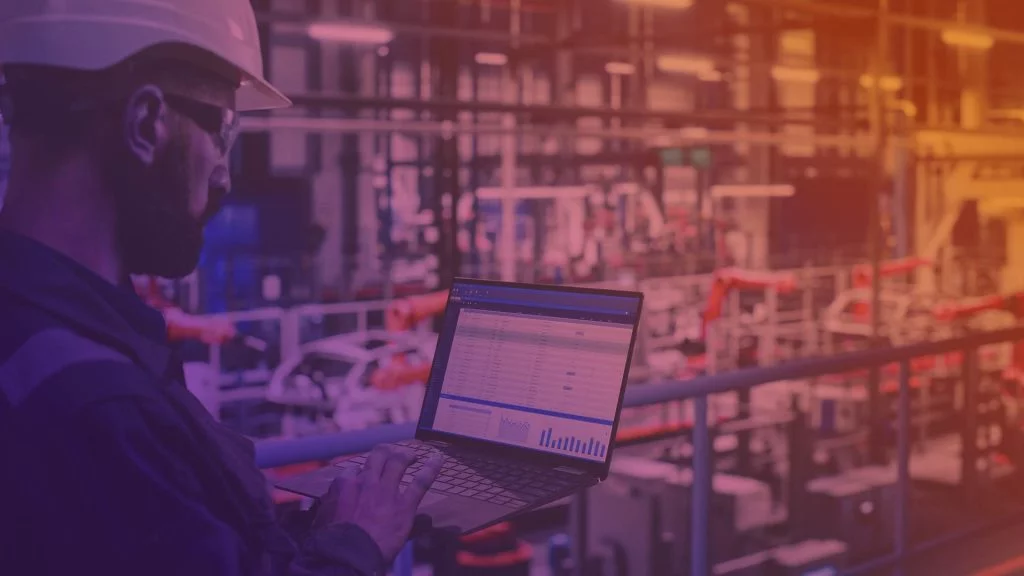
Analitica dei dati di produzione - Sfruttare le intuizioni con una piattaforma dati aziendale
Analisi dei dati di produzione: Unlocking Insights with an Enterprise Data Platform L'industria manifatturiera sta vivendo una trasformazione digitale, alimentata dalle grandi quantitร di dati generati in tutto il mondo.
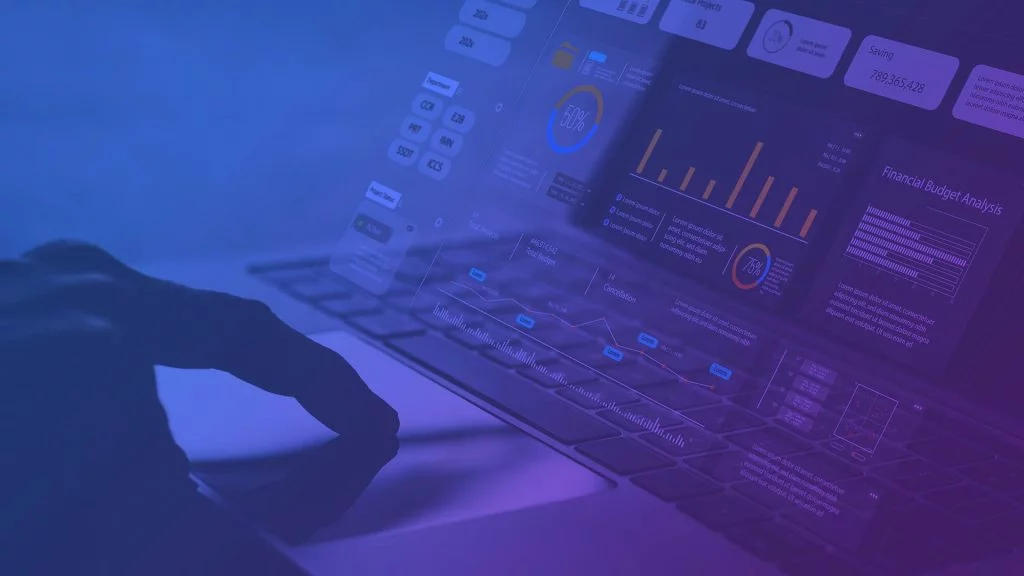
Metodi di integrazione e preparazione dei dati in fabbrica
I dati sono la chiave Nel settore manifatturiero, i dati vengono generati da una moltitudine di fonti, tra cui apparecchiature di produzione, sensori, sistemi ERP e controllo qualitร .